- Biodesign Academy
- Posts
- Rethinking Design When AI Enters the Lab
Rethinking Design When AI Enters the Lab
What Google’s Co-Scientist Means for Biodesign
Co-Scientist / Co-Designer: Your New Lab Partner (Who Already Has a Better H-Index)
Before we proceed, a quiet admission: Google’s Co-Scientist is frighteningly good.
It doesn’t sleep, doesn’t miss citations, and will almost certainly outrank me, and most of my colleagues, on Google Scholar. (Come to think of it, if it had its own ResearchGate profile, I suspect it would be bombarded with requests for full paper downloads and access 😉).
If academic tenure were open to non-carbon-based applicants, I’d be polishing my fallback CV. Probably a good thing I left academia when I did, eh?
So what is it, really?
Just because it was designed to assist scientific researchers, don’t think designers and design researchers are safe.
Systems like this are more than capable of surfacing entirely new design methods and generating speculative hypotheses in their own right—especially when design work involves system logic, material iteration, or protocol building.
Essentially, Co-scientist is:
A distributed team of agents (Generate, Reflect, Rank, etc.)
Designed to propose hypotheses, experiments, and literature syntheses
More like a design reasoning engine than a statistical model → It iterates, critiques, ranks, and refines—like a design team working through a brief, not a tool executing a command
Design (Not Discovery)
In February 2025, Google (quietly) unveiled its AI Co-Scientist, a multi-agent assistant for scientific research built on the Gemini 2.0 model.
It was pitched as a collaborator, one that could help generate hypotheses, design experiments, and surface insights buried in the literature.
The academic response? A mix of fascination and mild existential dread. Not surprising, really. Who wouldn't be nervous?
But biodesign doesn’t operate solely in this register. It is not only about knowing, but shaping, printing, and fabricating; not just understanding biology, but working with it as a material.
The arrival of a multi-agent AI collaborator invites deeper reflection. What kind of design logic does it bring into the lab? And what happens when that logic encounters the autonomy of living systems?
For biodesigners, this hits a nerve. Shiny technologies like AI are often lauded in scientific circles, while their knock-on effects on design workflows and protocols often go unexamined.
Yet these developments reshape how we design with life, its timing, temperament, and mutability.
Strategic Implications for Design Research
A Shift in How We Think and Work
For biodesign, this shift runs deep. Unlike industrial design or software UX, the iterative process in biodesign is often driven by the behaviour of living systems—systems whose rhythms, resistances, and trajectories unfold in ways we cannot fully predict or direct.
Will AI overwrite this complexity with premature clarity? I worry it might.
Take mycelium composites: their structural qualities depend on inconsistent variables like substrate, humidity, and microbial ecology.
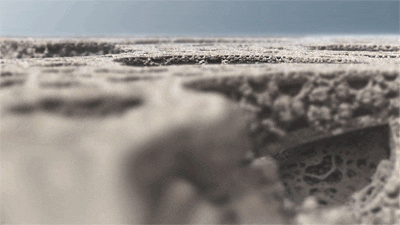
Gif by thefractalist on Giphy
My colleague once spent weeks tending to a particularly promising mycelium culture. No algorithm has ever felt the disappointment when your beautiful growth pattern suddenly decides to go rogue after two weeks of perfect behavior.
What if AI flattens this nuance into “optimal conditions” and steers us toward homogeneity?
Or bacterial cellulose: designers often tune texture and transparency via intuition, touch, smell. These properties emerge from process, not preset models. Would an AI ignore the artistry of fermentation?
Even algae systems, where pigmentation shifts with light, stress, or strange human interactions, defy formalisable inputs. Will Co-Scientist recognise these phenomena as signals or noise?
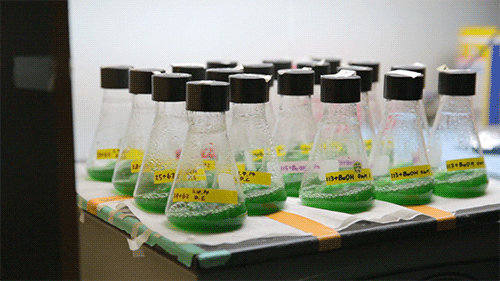
Gif by uofcalifornia on Giphy
When AI Starts Designing With You
These agents don’t just analyse data, they make calculated judgements. They reflect, evolve, and rank ideas. That sounds suspiciously like design thinking.
But design is also tacit. Much of it comes from standing over petri dishes, biocomposites, etc., and watching something behave or display oddly. From failure. From intuition.
If we reshape our briefs to fit the AI’s processing style, will we end up designing not for biology, but for computational convenience?
Imagine a team working on microbial biomineralisation. AI may rank “success” by mechanical strength, but the designer’s goal might be expressive material change under heat.
Or in scaffolding experiments: what if the magic lies not in optimising growth, but in observing how cells drift, resist, or collapse?
Living Systems and the Ethics of Delegated Intervention
Biodesign treats life as a partner, not just a medium. Bacteria, algae, cells, they co-shape outcomes.
With AI in the mix, are we tipping back toward control?
In kombucha leather production or pigment-producing microbes, unpredictability is not a flaw, it's the point.
Similarly, in interactive bio-HCI projects like biotic games, the joy often emerges from glitches, microbial randomness, or unexpected feedback loops. These aesthetic and behavioural artefacts become sources of play, speculation, and creative provocation.

If AI starts optimising these systems, we risk sanding down their expressive, embodied potential and silencing the microbial idiosyncrasies that make such systems meaningful.
We might be shifting from human-over-nature to human-plus-AI-over-nature. There's a real concern that the wonders of bio-agency will be ironed out by machine logic. These aren't just abstract worries; they speak to the core of what biodesign should be.
Safety in the Absence of Intuition
This is also a safety issue. Consider algae bioreactors used for photosynthetic patterning, or multi-strain microbes engineered for adaptive response. Such systems can behave unexpectedly, and only through embodied interaction do we understand when something is off. Can AI pick up on the whiff of fungal contamination in a petri dish, or some other funky smell of agar gone wrong?
The tactile knowledge, the olfactory warnings, the visual cues that experienced biodesigners rely on—these aren't easily quantified or taught to an AI. When we delegate intervention decisions to systems without intuition, we introduce new vulnerabilities into our work with living materials.
Towards a Different Kind of Integration
We don’t need to reject Co-Scientist. But we must re-frame how it fits within the craft of biodesign. Especially for projects involving fermented textiles, living colour systems, or bio-fabricated structures: the goal is not just output, but process.
Can we co-design with these agents, not just use them?
Should we build wet labs that accommodate contradiction, ambiguity, or pause?
Can AI become legible to biology, and to us?
Something to Consider
To labs, startups, and educators building the future of biodesign: don’t just adopt Co-Scientist. Interrogate it.
How does it shape your design stance toward biology?
Are your systems biologically responsive, or merely computationally elegant?
And when things go off-script, as they always do, will your team listen to the living system, or the ranking agent?
As we leave the lab (for a coffee break)…
In the end, maybe the most human thing we can do is resist the urge to be too efficient. In biodesign, the unexpected is often the most meaningful.
If Co-Scientist ends up with more citations, followers, and better funding metrics, fair play. But it still can’t watch cells grow, hesitate at the smell of bacterial cellulose, or make peace with an unruly phototactic microbe that refuses to glide elegantly.
And frankly, it’s not allowed to drink bad coffee in the lab at 9pm while rethinking everything, quietly fuming about your supervisor’s last-minute feedback, and wondering if this is all just a very expensive art degree in disguise. That bit’s still ours.
Practical information about Google Co-Scientist
Further reading here.
The official announcement from Google Research provides an overview of the Co-Scientist's architecture, purpose, and initial real-world applications. It explains how the system leverages a team of specialised AI agents to mimic scientific reasoning workflows, generating hypotheses, synthesising data, and proposing experimental designs.
The post also showcases early case studies, including collaborations with academic labs, and underscores the system's goal of becoming a true research collaborator rather than a mere tool.
You might need a Google account to sign up for early access. At the time of writing, it’s still in experimental preview, so not all features may be available immediately.
Enjoyed this post?
📩 Forward it to a curious lab mate
🧪 Stay tuned for Part II: What AI Means for Design Methods
🔖 Save it for your next team meeting
Looking for unbiased, fact-based news? Join 1440 today.
Join over 4 million Americans who start their day with 1440 – your daily digest for unbiased, fact-centric news. From politics to sports, we cover it all by analyzing over 100 sources. Our concise, 5-minute read lands in your inbox each morning at no cost. Experience news without the noise; let 1440 help you make up your own mind. Sign up now and invite your friends and family to be part of the informed.